C01 Elucidating Functional Roles of Nonlinear TD Errors Guided by Reinforcement Learning as Inference
Reinforcement learning has attracted attention as a model of biological decision making, and it is known that prediction errors of future rewards are strongly correlated with dopamine in the brain. However, recent research has reported that these two are not limited to a simple linear relationship, but rather encompass a variety of nonlinearities in combination. Unfortunately, the model that represents such nonlinearities are heuristically designed, and their theoretical justification is weak. It is also unclear what kind of nonlinearity is included, how it is distributed, and by what process it is acquired.
Therefore, this study theoretically derives the prediction errors with various nonlinearities in a new reinforcement learning theory based on “Control as Inference”. The functional roles of these nonlinearities are investigated through analyses that include not only the acquired behaviors but also the learning process. Afterwards, the parameters that determine them are systematized. A stochastic generative model of the parameters is designed with an updating principle using universal norms such as free energy. Using this new theory as a model, the actual brain activity data will be elaborately analyzed in collaboration with the members in this field.
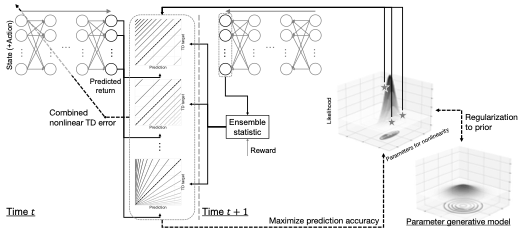
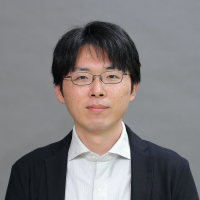
Principal Investigator: Taisuke Kobayashi
Assistant professor, National Institute of Informatics