C02 Building brain computational models for symbol sequence prediction
We use various symbol systems such as language, mathematical formulas, and music to communicate. Surprisal theory uses the probability of word occurrence in a given context to quantify the difficulty of sentence processing in natural language. With the development of large language models in recent years, it has become possible to compare these models with sentence processing in the human brain. Meanwhile, other methods such as encoding models and representational similarity analysis have been widely used to evaluate the relationship between large language models and brain activity, but their relationship with the surprisal theory remains unclear. In our research project, we will compare the effectiveness of surprisal theory and other methods in quantifying the relationship between large language models and the human brain. Using functional magnetic resonance imaging data with natural language and mathematical stimuli, we will calculate surprisal based on large language models. We will also perform encoding model and representational similarity analyses to predict brain activity from latent features extracted from large language models. We aim to compare the predictive accuracy of these different methods and identify the best method to explain the relationship between large language models and the human brain.
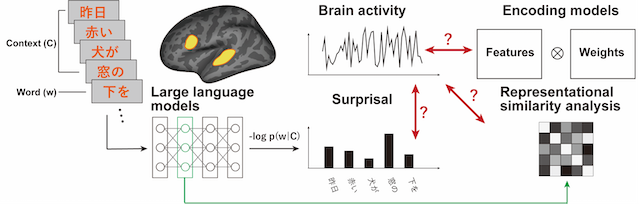
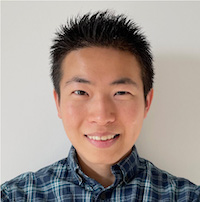